

For that reason, one usually tries to keep to simplified processes, still quite relevant.Ī Markov process is a process where all information that is used for predictions about the outcome at some time is given by one, latest observation. However, such a general situation becomes very cumbersome, and is almost hopeless to treat by any manageable formalism. The more observations we have made, the better we can predict the outcome at a later time. In general, that probability depends on what has been obtained in the previous observations. This means that, at each observation at a certain time, there is a certain probability to get a certain outcome. This comprises essentially everything we speak about. Basic references for this are Keizer, 1987 van Kampen, 1992 Zwanzig, 2001.Ī stochastic process means that one has a system for which there are observations at certain times, and that the outcome, that is, the observed value at each time is a random variable. We go on and now turn to stochastic processes, random variables that change with time. Understanding and modelling of biological noise, that plays an important role in cell fate decisions, environmental sensing and cell-cell communication, is another issue of main interest, as reported by Eldar and Elowitz in a recent review ( Eldar and Elowitz, 2010).Ĭlas Blomberg, in Physics of Life, 2007 18A Introduction: general account Just to cite a few advanced topics that are currently considered: models of passive and active transport in cells models of self-organization of cytoskeletal structures models for the interplay between diffusion and nonlinear chemical reactions. The relevance of noise and stochastic modelling to state-of-the-art molecular and cell biology is thus unquestionable. “The message that keeps being repeated is that the kinetics of biological processes at the intra-cellular level are stochastic, and that cellular function cannot be properly understood without building that stochasticity into in silico models” ( Wilkinson, 2012, Preface). Stochastic processes and biological/biochemical modelling are increasingly merging in recent years as technology has started giving real insight into intra-cellular processes: quantitative real-time imaging of expression at the single-cell level and improvement in computing technology are allowing modelling and stochastic simulation of such systems at levels of detail previously impossible. You can leave a response, or trackback from your own site.Maria Francesca Carfora, in Encyclopedia of Bioinformatics and Computational Biology, 2019 Future Directions/Closing Remarks You can follow any responses to this entry through the RSS 2.0 feed. January 20, 2014)įriday, December 20th, 20135:38 pmThis entry was posted on at and is filed under Uncategorized. Self study exercise problems (Assigned on Dec. Homework #4 (Unfinished problems in the midterm exam) Lecture 25: Hidden Markov Models (continued) Lecture 23: The Expectation-Maximization Algorithm Hidden Markov Models (HMMs) Lecture 22: Maximum-Likelihood Estimation Lecture 19: Conditional Expectations, MMSE, and Regression Analysis Lecture 18: Random Walks and Brownian Motion Lecture 17: Continuous-Time Markov Chains (CTMC)
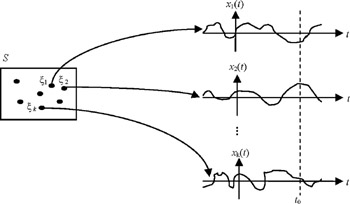
Lecture 16: Classification of States in a DTMC and Semi-Markov Processes Lecture 15: Discrete-Time Markov Chains (DTMC) Lecture 14: Applications of the K-L Expansion and the Poisson Process
#Random processes series
Lecture 13: Generalized Fourier Series Expansion: The Karhuenen-Loève Expansion Lecture 11: Introduction to Random Processes-cont’d Lecture 10: Limit Theorems-cont’d Introduction to Random Processes Lecture 9: Modes of Convergence-cont’d Limit Theorems Lecture 8: Inequalities and Bounds Modes of Convergence Lecture 7: Generating Functions and the Laplace Transform Lecture 6: Rayleigh, Rice and Lognormal Distributions Transform Methods and the Central Limit Theorem Lecture 5: Fundamental of Statistical Analysis, and Distributions Derived from Normal Distribution Lecture 4: More on Random Variables and Functions of Random Variables Lecture 1: Introduction: History and Overview Lecture 2: Probability and Random Variables Mark and William Turin (Cambridge University Press, 2012, 800 pages). The slides should be also useful to those who wish to study the subjects based on the textbook Probability, Random Processes and Statistical Analysis, by Hisashi Kobayashi, Brian L. I post here the lecture slides, hoping that they will be useful to other instructors who will teach similar courses. I taught the same course in the Fall 2012-13. I am currently teaching a graduate course “ ELE 525: Random Processes in Information Systems” at Princeton University on Mondays and Wednesdays in the Fall Semester 2013-14.
